The story so far...
As part of my MEng at Durham University (2018 - 2022), I performed an experiment in which I wanted to see if a deep learning (DL) model built for image recognition
could apply it's state-of-the-art architecture to the relatively underexplored problem of keystroke acoustic classification. This experiment began with a prompt
from my supervisor Ehsan Toreini, who had completed similar attacks in the past targeting the Enigma Machine.
Despite the existence of published work on the topic, this experiment would be the first attempt at exploring laptop keyboard accoustic attacks that prioritised
a DL architecture, used minimal off-the-shelf equipment and novelly used an image recognition model for signal analysis. This experiment later became my dissertation,
and following my graduation (and excellent editing by Maryam Mehrnezhad) became my first published paper,
titled: A Practical Deep Learning-Based Acoustic Side Channel Attack on Keyboards.
A day after publishing on Arxiv (a couple of weeks after publication in IEEE)
tweets began circulating about the paper, prompting small Cybersecurity blogs to feature
the paper and soon larger publications were chasing the tails of those blogs. Less than a day later, I was getting messages from friends saying things like "my Grandma saw you in the paper"
or "Elon Musk liked a tweet about you!". It was that day the interview requests and questions about the experiment and source code began... see below for the results!
While I am confident in saying this will be the last time my work gets seen to such an impressive degree. Thank you to everyone who kept me up to date,
and gave their support when it all got a bit overwhelming.
- Published: 07/2023
- Conference: 8th IEEE European Symposium on Security and Privacy
- Paper: Arxiv
- Dataset: GitHub
Press and TV
Below are a few of the mentions the paper has gotten in the press, keep checking back or follow the AltMetric to see updates!
- All
- News
- TV
- Radio/Podcast
Contact
Email:
LinkedIn:
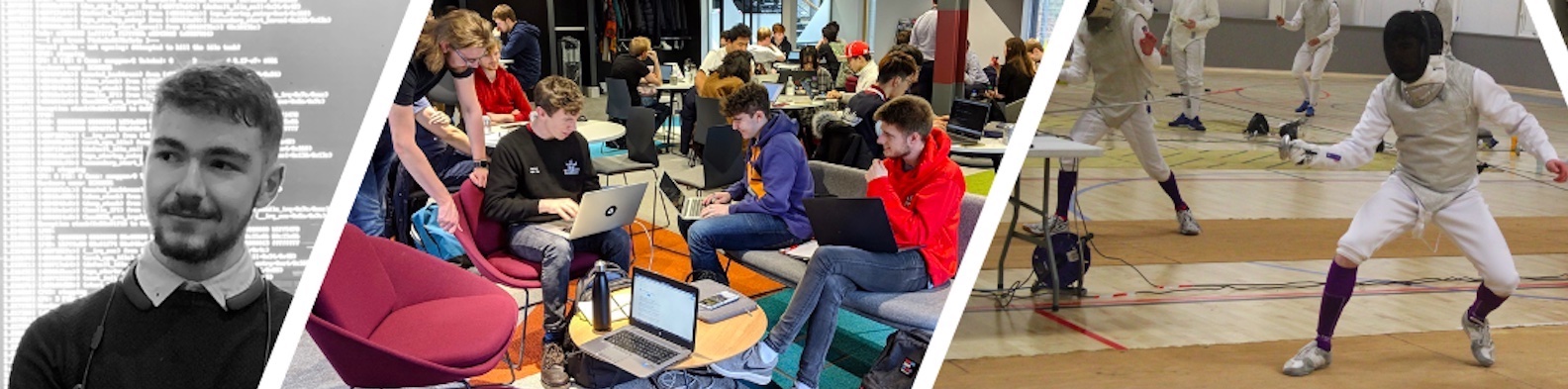